Research papers come out far too frequently for anyone to read them all. That’s especially true in the field of machine learning, which now affects (and produces papers in) practically every industry and company. This column aims to collect some of the more interesting recent discoveries and papers — particularly in, but not limited to, artificial intelligence — and explain why they matter.
This week we have a number of entries aimed at identifying or confirming bias or cheating behaviors in machine learning systems, or failures in the data that support them. But first a purely visually appealing project from the University of Washington being presented at the Conference on Computer Vision and Pattern Recognition.
They trained a system that recognizes and predicts the flow of water, clouds, smoke and other fluid features in photos, animating them from a single still image. The result is quite cool:
Why, though? Well, for one thing, the future of photography is code, and the better our cameras understand the world they’re pointed at, the better they can accommodate or recreate it. Fake river flow isn’t in high demand, but accurately predicting movement and the behavior of common photo features is.
An important question to answer in the creation and application of any machine learning system is whether it’s actually doing the thing you want it to. The history of “AI” is riddled with examples of models that found a way to look like they’re performing a task without actually doing it — sort of like a kid kicking everything under the bed when they’re supposed to clean their room.
This is a serious problem in the medical field, where a system that’s faking it could have dire consequences. And a study, also from UW, finds models proposed in the literature have a tendency to do this, in what the researchers call “shortcut learning.” These shortcuts could be simple — basing an X-ray’s risk on the patient’s demographics rather than the data in the image, for instance — or more unique, like relying heavily on conditions in the hospital its data is from, making it impossible to generalize to others.
The team found that many models basically failed when used on datasets that differed from their training ones. They hope that advances in machine learning transparency (opening the “black box”) will make it easier to tell when these systems are skirting the rules.
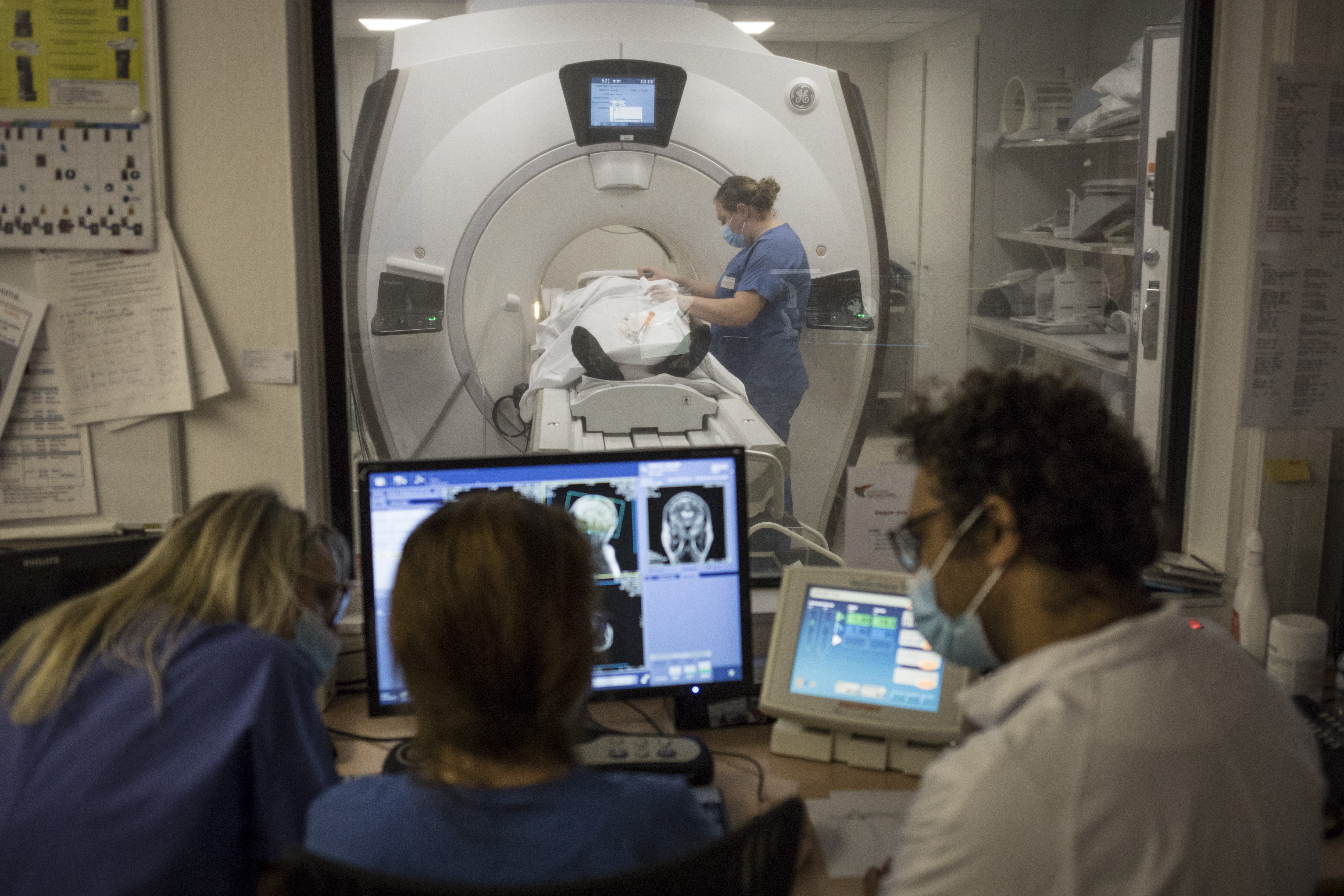
Image Credits: Siegfried Modola (opens in a new window) / Getty Images